14.03 Integrated Learning and Optimization for Mobility and Transportation Services (ILOMYTS)
The introduction of new business models in transportation is exposed to a large degree of uncertainty at the stage of design and operations due to limited available historical information. Therefore, integral parameters for product and process design need to be learned. Typically, forecasting and optimization are performed sequentially.
In this interdisciplinary project, we follow an integrated approach and build on concepts from datadriven optimization, stochastic programming and machine learning to develop decision support with the application to transportation and mobility, in particular for bike- and car-sharing. Based on bike- and car-sharing data from the cities of Copenhagen and Munich, exploration and exploitation methods and active learning concepts will be developed to support strategic and operational decision making with regard to capacity, inventory positioning and resource rebalancing under uncertainty in large systems with distributed (competitive) decision making. This integrated approach requires knowledge from the disciplines of transportation and management as well as from stochastic optimization and machine learning.
Team
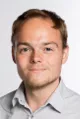
Project team leader
Dr. Dennis Prak
Chair of Logistics and Supply Chain Management | TUM
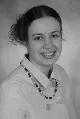
Doctoral researcher
Layla Martin
Chair of Logistics and Supply Chain Management

Doctoral Researcher
Daniele Gammelli
Transportmodelling | DTU
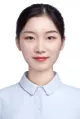
Doctoral researcher
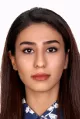
Doctoral Researcher
Melika Mohsenizadehkamou
Chair of Logistics and Supply Chain Management
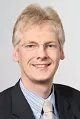
Principal Investigator
Professor Stefan Minner
Chair of Logistics and Supply Chain Management | TUM
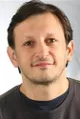
Principal Investigator
Professor Francisco Camara Pereira
Transportmodelling | DTU