MODA
Project: Transport Mode Detection and Analysis
Collaborating departments: TUM School of Engineering and Design; National Technical University of Ukraine - Igor Sikorsky Kyiv Polytechnic Institute (NTUU KPI)
Travel mode detection is a crucial task for transport demand management and understanding mobility patterns. Lack of data makes the mode detection task challenging to detect new transport modes and track trip chains. Thus, researchers and practitioners try to identify new data sources and plan optimal data collection infrastructure to develop advanced and robust models. In the MODA project, we will tackle travel mode detection from both the data as well as the modeling side. We aim to design and implement a simulation test bed to collect (generate) the mobility data covering new and traditional transport modes. We will design and test the opportunistic data collection framework to reflect the real-world challenges of data scarcity, sparsity, quality, and technical feasibility. Use of wavelet transformation and signal processing methods will be used to process and filter the data. We will propose and test state-of-the-art deep learning models to detect travel modes with high accuracy and robustness while addressing data quality issues. These models will be validated with respect to the simulation ground truth, as well as real-world data. Finally, models will be transferred to new contexts and study areas to tackle the lack of data. Thus, our proposal tackles the travel model detection holistically and has potential practical benefits to the transport research community. It provides a wider framework to apply mode detection using free and opportunistic data, assesses if these data are sufficient for the task, and investigate the limits of the data.
Yang, N., Lu, Q., Yamnenko, I., & Antoniou, C. (2024). Efficient Cloud-Sourced Transport mode detection using trajectory Data: a Semi-Supervised Asynchronous Federated Learning approach. In: IEEE Internet of Things Journal, 1. https://doi.org/10.1109/jiot.2024.3516695
Yang, N., Lu, Q. L., Lyu, C., & Antoniou, C. (2024). Transfer Learning for Transportation Demand Resilience Pattern Prediction Using Floating Car Data. In: Transportation Research Record. https://doi.org/10.1177/03611981241245681
Team
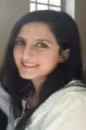
Coordinating Postdoc
Christelle Al Haddad
Chair of Transportation Systems Engineering | TUM
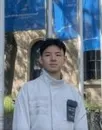
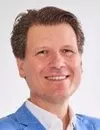
Principal Investigator
Professor Dr. Constantions Antoniou
Chair of Transportation Systems Engineering | TUM
Principal Investigator
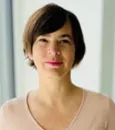
Professor Iuliia Yamnenko
National Technical University of Ukraine - Igor Sikorsky Kyiv Polytechnic Institute | NTUU KPI