LEADS
Project: Learning and Analyzing Discrete Geometric Structure in Statistical Models
Collaborating Departments: Department of Mathematics - Probability and Statistics (TUM) ; Department of Mathematics (Imperial)
In many scientific domains, highly multivariate data are collected to understand the behavior of systems with a large number of interacting units. In order to tractably represent patterns of stochastic dependence among these units, statistical models often employ discrete structure in the form of trees or, more generally, graphs and networks. This project develops new methods to learn the discrete structure from high-dimensional data, with a view towards applications in biology and a focus on efficient learning of large scale trees. Different algorithms will be developed under different hypotheses on the input data. In particular, the considered scenarios include problems with Gaussian and also explicitly non-Gaussian data as well as data coming from mixed observational and experimental settings. In applications such as genomic studies of rapidly mutating viruses, the analysis of tree/graph structure learned from many different individual data sets is crucial. To this end, the project also develops novel mathematical concepts and associated statistical methodology to analyze sets of tree/graph-valued data. Merging perspectives from tropical geometry and algebraic statistics, we will establish new definitions of statistically useful distance concepts and formal hypothesis tests that allow one to discern significant differences among groups of tree/graph-valued data.
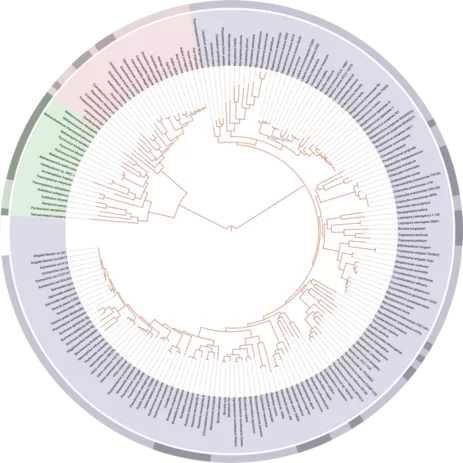
Our project (LEADS) is concerned with learning and analyzing discrete geometric structure in statistical models. The TUM Doctoral Researcher Daniele Tramontano started his work by focusing on statistical methods for causal structure learning in large-scale settings. The aim of this work is to achieve scalability by targeting only essential features that are captured by a tree structure. Specifically, Daniele has developed new methods for learning a polytree (a directed acyclic graph whose underlying skeleton is a tree). His first publication from summer 2022 takes up the setting of linear non-Gaussian models, and a second work that was submitted for publication is considering the case where data from different experimental settings are available. In subsequent work he will consider scenarios in which the variables are affected by measurement error. The Imperial Doctoral Researcher Roan Talbut has successfully passed her first-year PhD classes and her candidate exam (September 2022). In her research she has begun working on defining new distances between trees from different tree spaces, motivated by applications in phylogenetics. The foundational results have been obtained, and the work has already been presented at an academic conference. She is completing the manuscript for a submission to a Mathematical Biology journal. The first work has led to interesting optimization problems on the Tropical Projective Torus that are currently under investigation.
Daniele Tramontano; Anthea Monod; Mathias Drton (2022): Learning Linear Non-Gaussian Polytree Models.
Conference on Uncertainty in Artificial Intelligence 2022. https://www.auai.org/uai2022/accepted_papers
Daniele Tramontano, Leonard Waldmann, Mathias Drton, and Eliana Duarte (2023), Learning linear Gaussian polytree models with Interventions. SUBMITTED
Jakub Bober, Anthea Monod, Emil Saucan, Kevin N. Webster (2022): Rewiring Networks for Graph Neural Network Training Using Discrete Geometry, in: arXiv:2207.08026 [stat.ML].
Team
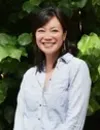
Principal Investigator (Imperial)
Prof. Dr. Anthea Monod
Assistant Professor in Mathematics | Imperial
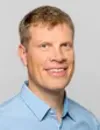
Principal Investigator (TUM)
Prof. Ph.D. Mathias Drton
Chair of Mathematical Statistics

Doctoral Candidate (Imperial)
Roan Talbut
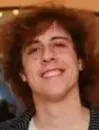