MoPreAnESD
Project: Modelling, Prediction and Anomaly detection of Earth Surface Dynamics
Collaborating Departments: Department of Mathematics, Statistics (Imperial); Remote Sensing Technology (TUM)
Geo-physical processes on Earth are governed primarily by complex, intertwined, and often non-stationary dynamical systems on various spatio-temporal scales. While it is possible to identify some isolated subprocesses, many of them interact with each other according to inscrutable and opaque causal patterns. Studying such processes by developing process-based models requires expert knowledge, but is limited by the availability of large and reliable data stocks, usually acquired in-situ at irregular spatial and temporal frequencies. With the launch and reliable operation of modern Earth observation satellite missions, it now became possible to monitor the Earth at various spatial and temporal scales with different sensor modalities. This coincides with the advent of powerful data-driven modelling techniques provided by the machine learning community and advances in stochastic modelling for efficient analysis of sequential measurements. If this expertise can be joined, together with modern big geospatial data management solutions, it seems possible to gain deeper and more detailed insights into the Earth system. We identified two sub-processes to investigate along the lines of these ideas, i.e., the seasonal growth of crops cultivated on the Earth's surface and the currents occurring in the oceans. Although occurring at different spatio-temporal scales, we believe they can be modelled in a generic data-driven way. To demonstrate the generalizability of our expected findings, we will apply them to model atmospheric processes assumed to drive the two originally considered systems.
Maja Schneider, Christian Marchington, Marco Körner (2022): Challenges and Opportunities of Large Transnational Datasets: A Case Study on European Administrative Crop Data, in: arXiv:2210.07178 [cs.DL].
Maja Schneider; Marco Körner (2022): Harnessing Administrative Data Inventories to Create a Reliable Transnational Reference Database for Crop Type Monitoring, in: IGARSS 2022 - 2022 IEEE International Geoscience and Remote Sensing Symposium.
Maja Schneider, Amelie Broszeit, Marco Krömer (2022): EUROCROPS: A Pan-European Dataset for Time Series Crop Type Classification, in: Proc. of the 2021 conference on Big Data from Space (BiDS’21), pp. 125-132. doi:10.2760/125905.
Dataset: EuroCrops: doi:10.5281/zenodo.6866846
Team
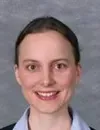
Principal Investigator (Imperial)
Professor Almut Veraart
Professor of Statistics
Department of Mathematics | Imperial
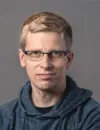
Principal Investigator (TUM)
PD Marco Körner
Adjunct Teaching Professor and Deputy Head
Chair of Remote Sensing Technology
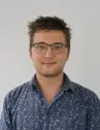
Doctoral Candidate (Imperial)
Dan Leonte
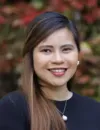